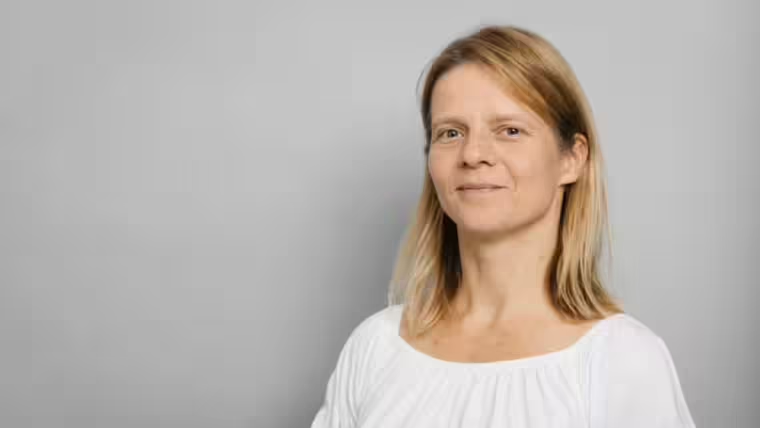
Published: | By: Lavinia Meier-Ewert; English translation by Gleb Chupakhin
Neuromorphic chips that process information like the human brain: This is the goal physicist Heidemarie Krüger (née Schmidt) is pursuing with her Dresden-based startup "Techifab." The researcher from Friedrich Schiller University Jena and the Leibniz Institute for Photonic Technologies is developing a technology that processes and stores information directly at its point of origin—without the energy-intensive data transfer between processor and memory. Together with her team, Krüger is developing memristor-based components that aim to set new standards in energy efficiency and computing power. This real-time-capable and resource-efficient technology could, for example, support self-driving cars and industrial facilities. "Our goal is to use the brain as a model to create a technology that makes complex decisions logically and traceably with minimal energy consumption," says Heidemarie Krüger.
The heart of the system: memristors with memory and the ability to learn
The neuromorphic chip is based on memristors—components that function similarly to synapses in the brain. They not only store information but can also process it simultaneously. While conventional computers constantly exchange data between memory and processor, this technology works locally. This significantly reduces energy losses and allows for fast, decentralized data analysis.
"A key difference is the ability of memristors to process continuous intermediate states—not just '0' and '1', but also values in between," explains Krüger. This flexible data processing opens up new possibilities for algorithms that emulate neural networks. Applications range from predictive machine maintenance to real-time analyses in safety-critical areas such as autonomous driving.
From lab discovery to industrial application
The path to this development began with an accidental discovery in the lab in 2011: During a material analysis, Krüger's team observed a characteristic "loop" curve—an indication of the behavior of a memristor with hysteretic memristance. This property enables the component to "remember" previous computations and thus perform complex calculations directly.
This led to the idea of developing artificial synapses from a material combination of bismuth and iron oxide. To turn these artificial synapses into a functional chip, the startup received multi-million euro funding from the German Federal Agency for Breakthrough Innovation (SPRIN-D). "We were able to show that these artificial synapses can efficiently handle even complex computational tasks like matrix multiplications," reports Krüger. These calculations, for example, form the basis for training many AI applications and image processing algorithms. In January 2025, the news magazine Der Spiegel will report on how Krüger’s technology could set new standards in the field of energy-efficient data processing.
Technology with potential for edge computing
The architecture of memristors allows data to be processed directly at the source—a key component for so-called edge computing, where data doesn’t need to be transferred to central cloud systems. "This means more security and independence, as sensitive data stays local," emphasizes Krüger. In industrial sensing, this could be a major advantage for capturing early signs of wear and preventing failures.
In initial pilot projects, Krüger's team is already testing the technology under real-world conditions together with the Technical University of Freiberg. It was shown that the neuromorphic chip can reliably detect even the smallest changes and predict wear patterns accurately.
A sustainable path to energy-efficient AI systems
While traditional processors require more and more transistors to handle the growing data flood, conventional chip design is reaching physical and energy limits. Neuromorphic approaches combine memory and processing units, reducing energy consumption and significantly expanding the potential for AI systems.
"Our goal is not just to analyze datasets but also to learn to recognize patterns and respond flexibly to new situations—without a constant connection to external data centers," explains Krüger. In the future, this technology could help make data centers more energy-efficient and enable AI applications to be developed with significantly lower resource requirements.
Krüger’s current prototype contains 32 memristors. In the next development stage, this number will increase to over 200 to model complex neural networks and enable new applications in autonomous systems.
Original Publications:
Y. Shuai, S. Zhou, D. Bürger, M. Helm, H. Schmidt: Nonvolatile bipolar resistive switching in Au/BiFeO3/Pt, J. Appl. Phys. 109, 124117 (2011), Appl. Phys. 109, 124117 (2011), https://doi.org/10.1063/1.3601113External link
H. Schmidt: Prospects for memristors with hysteretic memristance as so-far missing core hardware element for transfer-less data computing and storage, J. Appl. Phys. 135, 200902 (2024), https://doi.org/10.1063/5.0206891External link